Dealing with the myriads of digital information that flow throughout a business’s operations on a daily basis is no easy feat. As such, organizational leaders are continuously looking for ways to better manage it all and are considering data engineering services that can help.
Many are eager to leverage the data their business comes into contact with but may be unsure how exactly it can be done. After all, you can have an inkling about the usefulness of certain digital information but not have the resources to deal with thorough data analysis just yet. If that is the case, data lakes might be optimal for your company to implement.
However, prior to starting a new IT project of this kind, it’s a good idea for corporate leaders to familiarize themselves with data lake architecture. That way, you’ll be more in the loop during discussions with the development team.
Today, we’ll go over some of the following key details pertaining to data lake structure:
Let’s get into it.
Why Implement Data Lakes?
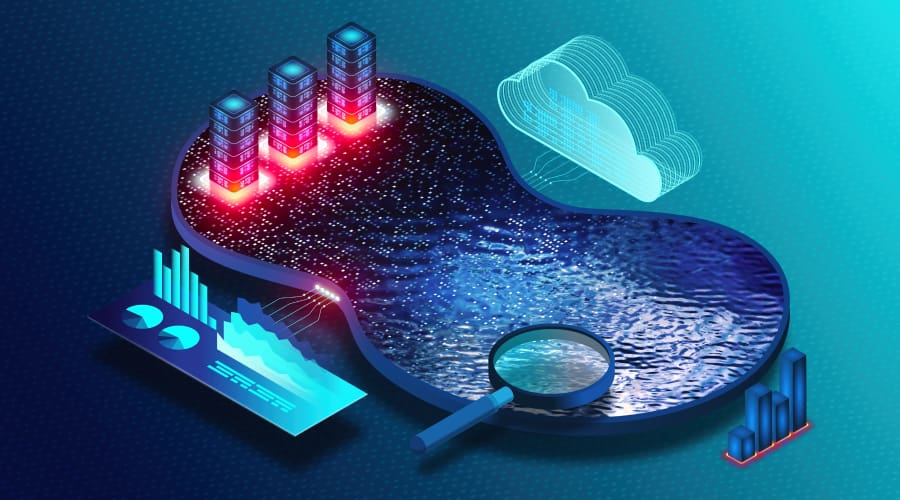
Let’s get the basics out of the way first and discuss the definition of data lakes. In short, they are centralized repositories for storing structured and unstructured digital information businesses collect from disparate sources.
Data lakes can store valuable details from web-based solutions, social media, mobile apps, IoT devices, and the like. Unlike data warehouses, these systems store information in its native state until it is retrieved for further processing.
Discover the Difference Between Data Lakes and Data Warehouses
There are many advantages of data lakes. From simplifying your data management practices and increasing analytical efficiency to reducing costs and facilitating data security, there’s no shortage of reasons for incorporating these platforms into your IT infrastructure.
Types of Data Lakes
Similar to enterprise data warehouses, data lakes can be implemented via the cloud or on-premises. Before we go deeper into the architecture of these solutions, let’s quickly go over how the two types of data lakes differ.
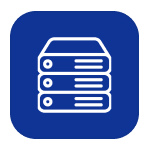
On-Premises
On-premises deployment requires that you buy and run the needed data lake software and hardware tools yourself or outsource these tasks to an outside vendor. Either way, it’s up to you to ensure that everything is running smoothly.
Read up on the Best Practices for Outsourcing Software Development
As you can imagine, this approach can be quite costly as you need to not only buy all of the software and hardware but also acquire IT specialists to manage and maintain the data lake. Moreover, as the digital information accumulates, you’ll need to migrate to a bigger system, which might be challenging given the existing hardware you’ve got.
Despite these drawbacks, many still opt for on-premises data lakes as they allow the company to retain control over the data and provide better security. Plus, thanks to no network latency, operations are much more efficient.
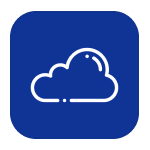
Cloud-Based
Cloud data lakes services have been gaining traction in recent years as businesses look for scalability, lower costs, and easy-to-manage maintenance. With this type of deployment, you get access to the system over the Internet as it will run on hardware and software of an outside supplier.
Popular examples of cloud-based data lakes service providers include Amazon’s S3 and Microsoft’s Azure Data Lake. In terms of payment, you’ll most likely be charged through a pay-as-you-go subscription that will allow you to scale up or down easily by increasing cloud storage capacity.
Find out how Velvetech carried out Cloud-to-Cloud Migration from Azure to AWS
The main issues you might run into with these types of data lakes are latency and security. Of course, popular providers have a good track record of ensuring efficiency and data safety but since everything is done over the Internet, the risk of leaks and latency is higher.
Data Lake Architecture Elements
Data lakes are often thought of as single repositories that simply accumulate digital information until it’s time for it to be used. However, the reality is that data storage within the lake can be divided into distinct layers or tiers.
In fact, layering the data lake will reduce the likelihood of it turning into a “data swamp” as the digital information is cataloged and indexed properly. Hence, let’s go through the key layers of data lake design so that you have an overview of what constitutes each one. That way, you’ll know what to expect during discussions with the IT team.
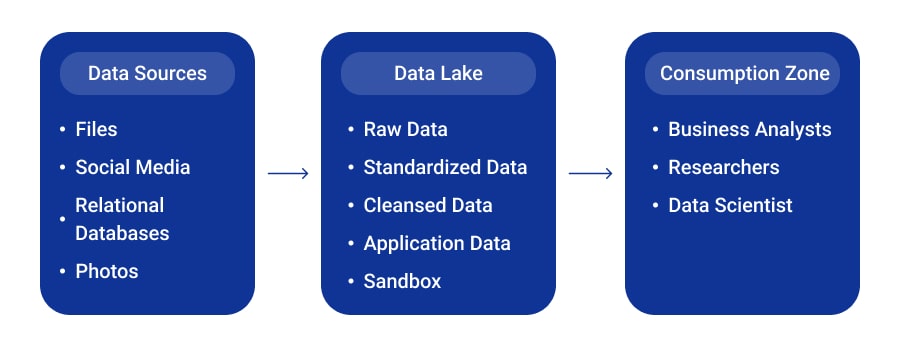
Data Ingestion Layer
As you may have already gathered, everything starts from the ingestion of raw digital information into the data lake. Through API connectors, data can be ingested in real-time or in batches from various sources like website analytics, CRM platforms, social media, wearables, and other tools. At this stage, no structuring or modification occurs, everything is simply extracted, loaded into the system, and organized in a logical folder structure.
Typically, the raw data can be divided by subject area, data source, and date of ingestion. This kind of a division allows for some degree of systematization and helps avoid too much chaos within the lake.
Standardized Data Layer
This data tier isn’t always implemented, but when you expect the data lake to grow quickly, it’s a good idea to include it. The main goal of the standardized data layer is to boost the transfer efficiency from a raw state to cleansed.
Here, unlike in the previously discussed layer, the data is stored in a format that is best fit for further cleansing. For example, it might be divided into more specific categories.
Cleansed Data Layer
The cleansed data tier, as the name suggests, is all about cleansed or curated data sets. Typically, at this level, the purpose of the acquired digital information is already known and the majority of cleansing or transformations has taken place.
As you can imagine, this might be the most complicated part of a data lake as the information is already on its way to being analyzed. Of course, if you don’t yet know what to do with the stored data, there is no use in focusing on this tier.
Application Data Layer
This element of data lake architecture is also known as the production or secure layer. It sources digital information from the cleansed tier, keeping the structure the same, and enforces any required business logic.
If you want to run machine learning algorithms or analytical tools within your data lake, it would most likely happen in this tier.
Sandbox Data Layer
The sandbox data tier can be considered optional within the data lake architecture as it is meant for complex data science or analytical work. Concretely, specialists may run experiments and manipulate enormous volumes of data within the sandbox as they look for hidden patterns or correlations.
Additionally, if you ever want to enrich your digital information with data from an external Internet source, this will be the place to do it.
Data Lake Architecture Tips
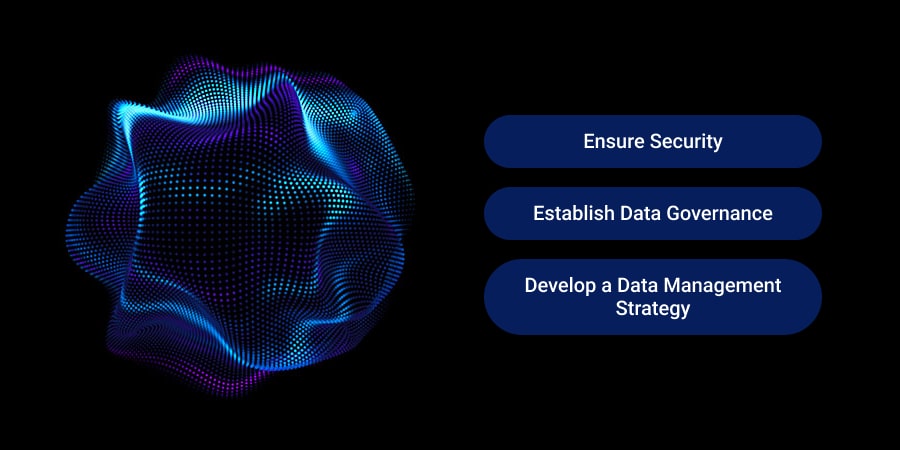
Now that we’ve gone over the key elements of enterprise data lake architecture, let’s briefly talk about some tips that you’ve got to keep in mind when pursuing the implementation of these solutions.
Ensure Security
As more and more digital information floods the business world, ensuring security becomes crucial. Thus, you’ve got to keep data safety top of mind when implementing every layer of the data lake.
Preventing unauthorized access and having measures in place in case of system failure or data breaches is imperative for the long-term success of data lakes. So, prior to development, consider spending some time laying out the security measures your system should possess.
Establish Data Governance
If you don’t want your data lake to turn into a disorganized swamp, it’s not only vital to catalog what is within the system but also keep track of how the digital information is managed and who is in charge of what. This can be done by establishing data governance.
Specifically, it’s a good idea to document internal policies and processes that cover how and by whom data-related activities are managed. That way, you’ll always have guidelines to refer to and can be confident that data integrity, quality, and security is continuously maintained.
Develop a Data Management Strategy
Lastly, for the data lake to fit in nicely within your organization’s IT structure, consider developing a comprehensive data management strategy. In fact, you may have already done it and ended up pursuing the implementation of this system after identifying what the company was missing.
Either way, don’t forget to note down the data lake’s role within your enterprise and make sure you take a well-thought-out approach to data management. It will definitely help truly reap the rewards of digital initiatives.
Get Started With Data Lakes
Like we said at the beginning of this post, managing digital information isn’t easy. Especially when you have seemingly endless amounts of it. Luckily, data lakes are here to help and you don’t even need to deal with developing their entire architecture yourself.
If you are not sure where to get started with data management or are looking for external specialists to help you on your data lake implementation journey — don’t hesitate to contact Velvetech.
We have an expert team that can seamlessly deliver top-notch data engineering services and help you step up your digital information storage game. Simply reach out via the form below and we’ll get back to you as soon as possible.